Data Visualization with Pandas and Matplotlib¶
# import library
import pandas as pd
import matplotlib.pyplot as plt
# display plot in the notebook
%matplotlib inline
# set figuresize and fontsize
plt.rcParams['figure.figsize'] = (8,6)
plt.rcParams['font.size'] = 14
# read data
drink_cols = ["country", 'beer', 'spirit', 'wine', 'liters', 'continent']
drinks = pd.read_csv("../data/drinks.csv", header=0, names=drink_cols, na_filter=False)
Data Exploration¶
# examine first few rows
drinks.head()
country | beer | spirit | wine | liters | continent | |
---|---|---|---|---|---|---|
0 | Afghanistan | 0 | 0 | 0 | 0.0 | AS |
1 | Albania | 89 | 132 | 54 | 4.9 | EU |
2 | Algeria | 25 | 0 | 14 | 0.7 | AF |
3 | Andorra | 245 | 138 | 312 | 12.4 | EU |
4 | Angola | 217 | 57 | 45 | 5.9 | AF |
# observations and columns
drinks.shape
(193, 6)
# data structure
drinks.info()
<class 'pandas.core.frame.DataFrame'>
RangeIndex: 193 entries, 0 to 192
Data columns (total 6 columns):
# Column Non-Null Count Dtype
--- ------ -------------- -----
0 country 193 non-null object
1 beer 193 non-null int64
2 spirit 193 non-null int64
3 wine 193 non-null int64
4 liters 193 non-null float64
5 continent 193 non-null object
dtypes: float64(1), int64(3), object(2)
memory usage: 9.2+ KB
# numerical summary
drinks.describe()
beer | spirit | wine | liters | |
---|---|---|---|---|
count | 193.000000 | 193.000000 | 193.000000 | 193.000000 |
mean | 106.160622 | 80.994819 | 49.450777 | 4.717098 |
std | 101.143103 | 88.284312 | 79.697598 | 3.773298 |
min | 0.000000 | 0.000000 | 0.000000 | 0.000000 |
25% | 20.000000 | 4.000000 | 1.000000 | 1.300000 |
50% | 76.000000 | 56.000000 | 8.000000 | 4.200000 |
75% | 188.000000 | 128.000000 | 59.000000 | 7.200000 |
max | 376.000000 | 438.000000 | 370.000000 | 14.400000 |
Histogram: show the distribution of a numerical variable¶
# sort the beer columns and split it into 3 groups
drinks.beer.sort_values().values
array([ 0, 0, 0, 0, 0, 0, 0, 0, 0, 0, 0, 0, 0,
0, 0, 1, 1, 1, 1, 2, 3, 5, 5, 5, 5, 5,
6, 6, 6, 6, 8, 8, 8, 9, 9, 9, 9, 12, 13,
15, 15, 16, 16, 17, 18, 19, 19, 20, 20, 21, 21, 21,
21, 22, 23, 25, 25, 25, 25, 26, 28, 31, 31, 31, 31,
32, 32, 34, 36, 36, 36, 37, 42, 42, 43, 44, 45, 47,
49, 51, 51, 52, 52, 52, 53, 56, 56, 57, 58, 60, 62,
62, 63, 64, 69, 71, 76, 76, 77, 77, 77, 78, 79, 82,
82, 85, 88, 89, 90, 92, 93, 93, 98, 99, 102, 105, 106,
109, 111, 115, 120, 122, 124, 127, 128, 130, 133, 140, 142, 143,
144, 147, 149, 149, 152, 157, 159, 162, 163, 167, 169, 171, 173,
185, 188, 192, 193, 193, 194, 194, 196, 197, 199, 203, 206, 213,
217, 219, 224, 224, 225, 230, 231, 233, 234, 236, 238, 240, 245,
245, 247, 249, 251, 261, 263, 263, 270, 279, 281, 283, 284, 285,
295, 297, 306, 313, 333, 343, 343, 346, 347, 361, 376])
# compare with histogram
drinks.beer.plot(kind="hist", bins=3);
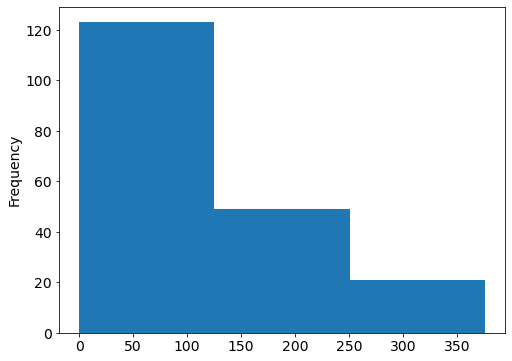
# try more bins
drinks.beer.plot(kind="hist", bins=20);
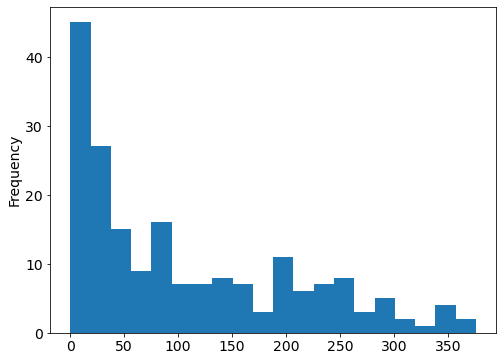
# add title and labels
drinks.beer.plot(kind="hist", bins=20, title="Histogram of Beer Servings")
plt.xlabel("Beer Survings")
plt.ylabel("Frequency")
# show plot
plt.show()
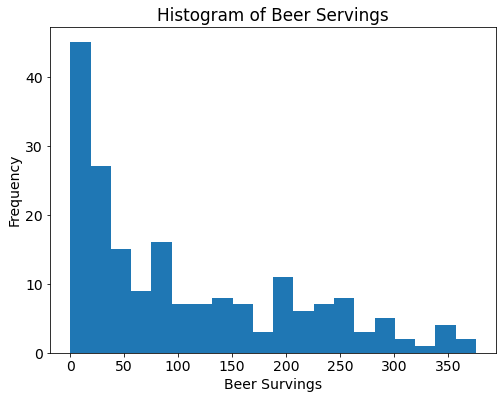
# compare with density plot(smooth version of a histogram)
drinks.beer.plot(kind="density", xlim=(0, 500));
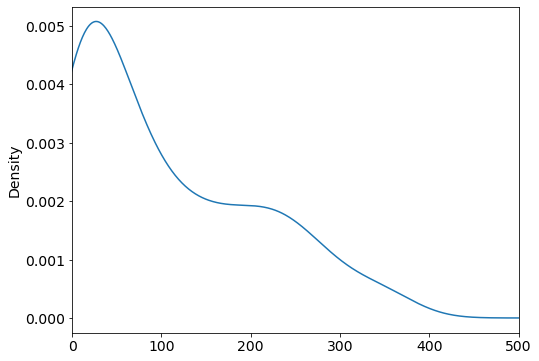
Scatter Plot: show the relationship between two numerical variables¶
# select the beer and wine columns and sort by beer
drinks[["beer", "wine"]].sort_values(by="beer").values
array([[ 0, 0],
[ 0, 74],
[ 0, 0],
[ 0, 0],
[ 0, 0],
[ 0, 0],
[ 0, 0],
[ 0, 0],
[ 0, 0],
[ 0, 0],
[ 0, 0],
[ 0, 0],
[ 0, 0],
[ 0, 0],
[ 0, 0],
[ 1, 7],
[ 1, 1],
[ 1, 4],
[ 1, 1],
[ 2, 0],
[ 3, 1],
[ 5, 0],
[ 5, 0],
[ 5, 16],
[ 5, 1],
[ 5, 0],
[ 6, 1],
[ 6, 0],
[ 6, 1],
[ 6, 9],
[ 8, 0],
[ 8, 1],
[ 8, 1],
[ 9, 2],
[ 9, 0],
[ 9, 7],
[ 9, 0],
[ 12, 10],
[ 13, 0],
[ 15, 3],
[ 15, 1],
[ 16, 5],
[ 16, 0],
[ 17, 1],
[ 18, 0],
[ 19, 32],
[ 19, 2],
[ 20, 0],
[ 20, 31],
[ 21, 11],
[ 21, 11],
[ 21, 5],
[ 21, 1],
[ 22, 1],
[ 23, 0],
[ 25, 8],
[ 25, 14],
[ 25, 2],
[ 25, 7],
[ 26, 4],
[ 28, 21],
[ 31, 128],
[ 31, 6],
[ 31, 10],
[ 31, 1],
[ 32, 4],
[ 32, 1],
[ 34, 13],
[ 36, 19],
[ 36, 5],
[ 36, 1],
[ 37, 7],
[ 42, 2],
[ 42, 7],
[ 43, 0],
[ 44, 1],
[ 45, 0],
[ 47, 5],
[ 49, 8],
[ 51, 20],
[ 51, 7],
[ 52, 2],
[ 52, 149],
[ 52, 26],
[ 53, 2],
[ 56, 140],
[ 56, 1],
[ 57, 1],
[ 58, 2],
[ 60, 11],
[ 62, 18],
[ 62, 123],
[ 63, 9],
[ 64, 4],
[ 69, 2],
[ 71, 1],
[ 76, 8],
[ 76, 9],
[ 77, 8],
[ 77, 16],
[ 77, 1],
[ 78, 1],
[ 79, 8],
[ 82, 9],
[ 82, 0],
[ 85, 237],
[ 88, 0],
[ 89, 54],
[ 90, 2],
[ 92, 233],
[ 93, 5],
[ 93, 1],
[ 98, 18],
[ 99, 1],
[102, 45],
[105, 24],
[106, 86],
[109, 18],
[111, 1],
[115, 220],
[120, 11],
[122, 51],
[124, 12],
[127, 370],
[128, 7],
[130, 172],
[133, 218],
[140, 9],
[142, 42],
[143, 36],
[144, 16],
[147, 4],
[149, 120],
[149, 11],
[152, 186],
[157, 51],
[159, 3],
[162, 3],
[163, 21],
[167, 8],
[169, 129],
[171, 71],
[173, 35],
[185, 280],
[188, 7],
[192, 113],
[193, 9],
[193, 221],
[194, 339],
[194, 32],
[196, 116],
[197, 7],
[199, 28],
[203, 175],
[206, 45],
[213, 74],
[217, 45],
[219, 195],
[224, 59],
[224, 278],
[225, 81],
[230, 254],
[231, 94],
[233, 78],
[234, 185],
[236, 271],
[238, 5],
[240, 100],
[245, 312],
[245, 16],
[247, 73],
[249, 84],
[251, 190],
[261, 212],
[263, 97],
[263, 8],
[270, 276],
[279, 191],
[281, 62],
[283, 127],
[284, 112],
[285, 18],
[295, 212],
[297, 167],
[306, 23],
[313, 165],
[333, 3],
[343, 56],
[343, 56],
[346, 175],
[347, 59],
[361, 134],
[376, 1]])
# comapre with scatter plot
drinks.plot(kind="scatter", x="beer", y="wine");
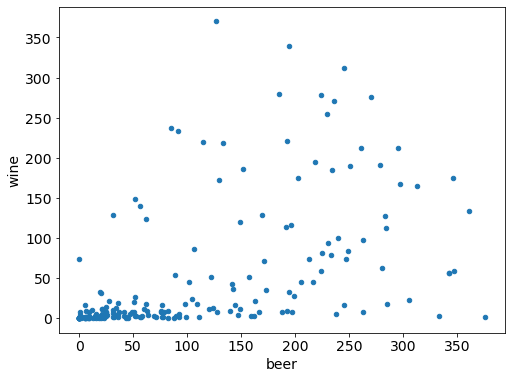
# add transparency
drinks.plot(kind='scatter', x="beer", y="wine", alpha=0.3);
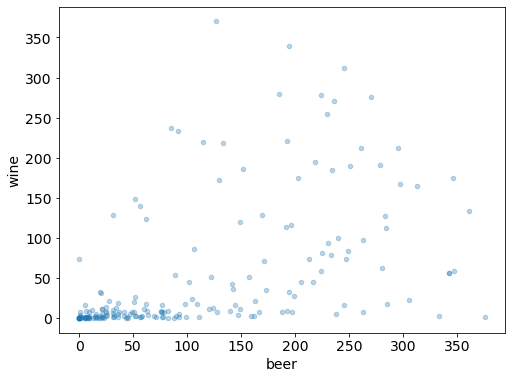
# vary point color by spirit servings
drinks.plot(kind="scatter", x="beer", y="wine", c="spirit", colormap="Blues");
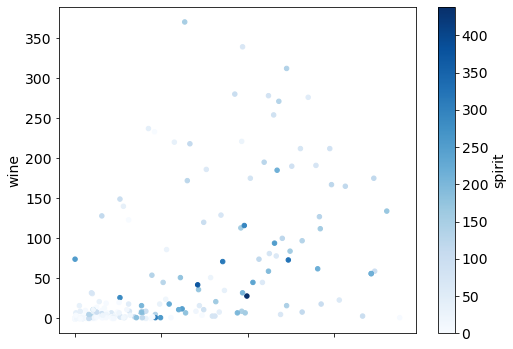
# scatter matrix of 3 numerical columns
pd.plotting.scatter_matrix(drinks[['beer', 'wine', 'spirit']]);
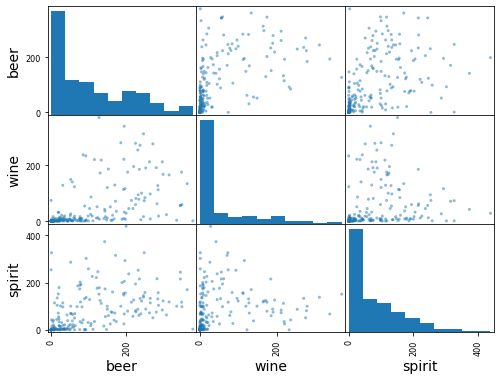
# increase figure size
# scatter matrix of 3 numerical columns
pd.plotting.scatter_matrix(drinks[['beer', 'wine', 'spirit']], figsize=(10,8));
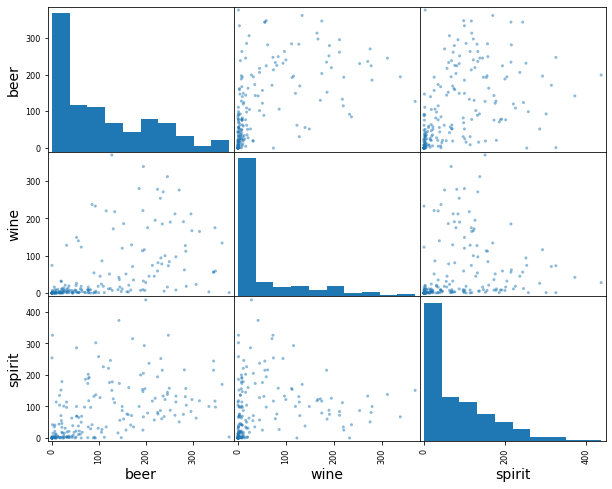
Bar Plot: show a numerical comparison across different categories¶
# count the number of countries in each continent
drinks.continent.value_counts()
AF 53
EU 45
AS 44
NA 23
OC 16
SA 12
Name: continent, dtype: int64
# compare with bar plot
drinks.continent.value_counts().plot(kind="bar");
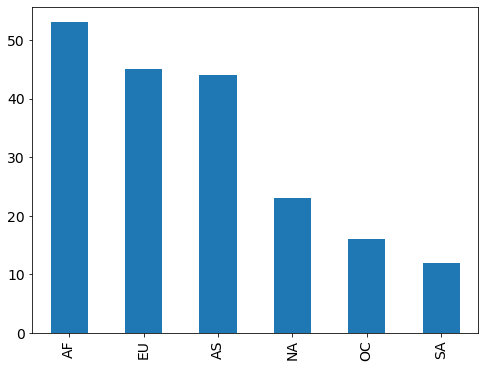
# calculate the mean alcohol amounts for each continent
drinks.groupby('continent').mean()
beer | spirit | wine | liters | |
---|---|---|---|---|
continent | ||||
AF | 61.471698 | 16.339623 | 16.264151 | 3.007547 |
AS | 37.045455 | 60.840909 | 9.068182 | 2.170455 |
EU | 193.777778 | 132.555556 | 142.222222 | 8.617778 |
NA | 145.434783 | 165.739130 | 24.521739 | 5.995652 |
OC | 89.687500 | 58.437500 | 35.625000 | 3.381250 |
SA | 175.083333 | 114.750000 | 62.416667 | 6.308333 |
# side-by-side bar plots
drinks.groupby('continent').mean().plot(kind='bar');
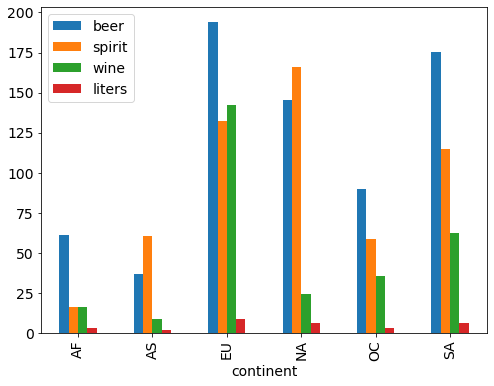
# drop the liters column
drinks.groupby('continent').mean().drop('liters', axis=1).plot(kind='bar');
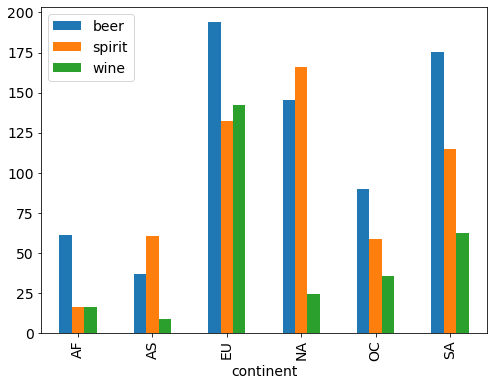
# stacked bar plots
drinks.groupby('continent').mean().drop('liters', axis=1).plot(kind='bar', stacked=True);
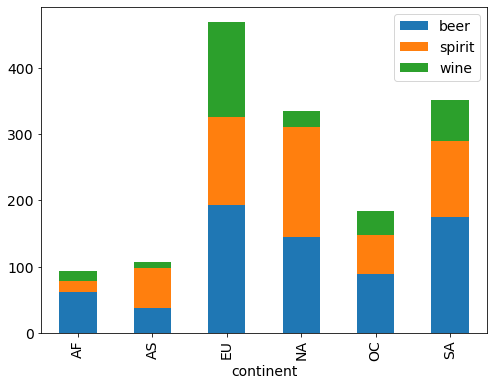
Box Plot: show quartiles (and outliers) for one or more numerical variables¶
Five-Number Summary
min = minimum value
5% = first quartile (Q1) = median of the lower half of the data
50% = second quartile (Q2) = median of the data
75% = third quartile (Q3) = median of the upper half of the data
max = maximum value (More useful than mean and standard deviation for describing skewed distributions)
Interquartile Range (IQR) = Q3 - Q1
Outliers
below Q1 - 1.5 * IQR
above Q3 + 1.5 * IQR
# sort the spirit column
drinks.spirit.sort_values().values
array([ 0, 0, 0, 0, 0, 0, 0, 0, 0, 0, 0, 0, 0,
0, 0, 0, 0, 0, 0, 0, 0, 0, 0, 1, 1, 1,
1, 1, 1, 1, 1, 1, 2, 2, 2, 2, 2, 2, 2,
3, 3, 3, 3, 3, 3, 3, 3, 4, 4, 4, 5, 5,
6, 6, 6, 7, 9, 11, 11, 12, 13, 15, 15, 16, 16,
18, 18, 18, 18, 19, 21, 21, 22, 22, 25, 25, 27, 29,
31, 31, 34, 35, 35, 35, 35, 38, 39, 41, 41, 42, 42,
44, 46, 50, 51, 55, 56, 57, 60, 61, 63, 63, 65, 67,
68, 69, 69, 69, 71, 71, 72, 74, 75, 76, 76, 79, 81,
84, 87, 87, 88, 97, 97, 98, 98, 100, 100, 100, 100, 101,
104, 104, 112, 114, 114, 114, 117, 117, 118, 118, 122, 122, 124,
126, 128, 131, 132, 133, 133, 135, 137, 138, 145, 147, 151, 152,
154, 156, 157, 158, 160, 170, 173, 173, 176, 178, 179, 186, 189,
192, 194, 200, 202, 205, 215, 215, 216, 221, 226, 237, 244, 246,
252, 254, 258, 286, 293, 302, 315, 326, 326, 373, 438])
# show five-number summary of spirit
drinks.spirit.describe()
count 193.000000
mean 80.994819
std 88.284312
min 0.000000
25% 4.000000
50% 56.000000
75% 128.000000
max 438.000000
Name: spirit, dtype: float64
# compare with boxplot
drinks.spirit.plot(kind='box');
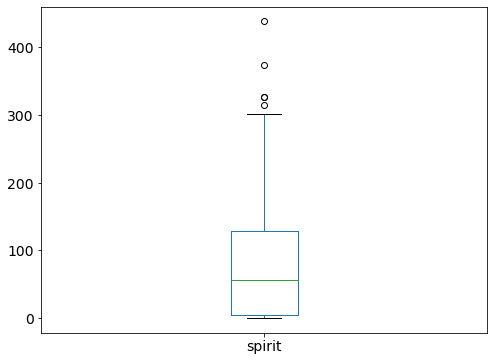
# include multiple variables
drinks.drop('liters', axis=1).plot(kind='box');
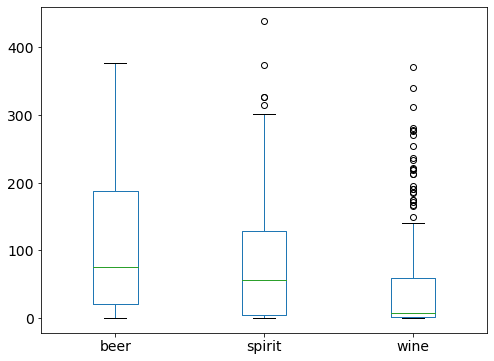
Line Plot: show the trend of a numerical variable over time¶
# read ufo data
ufo = pd.read_csv("../data/ufo.csv")
ufo['Time'] = pd.to_datetime(ufo.Time)
ufo['Year'] = ufo.Time.dt.year
# examine first few rows
ufo.head()
City | Colors Reported | Shape Reported | State | Time | Year | |
---|---|---|---|---|---|---|
0 | Ithaca | NaN | TRIANGLE | NY | 1930-06-01 22:00:00 | 1930 |
1 | Willingboro | NaN | OTHER | NJ | 1930-06-30 20:00:00 | 1930 |
2 | Holyoke | NaN | OVAL | CO | 1931-02-15 14:00:00 | 1931 |
3 | Abilene | NaN | DISK | KS | 1931-06-01 13:00:00 | 1931 |
4 | New York Worlds Fair | NaN | LIGHT | NY | 1933-04-18 19:00:00 | 1933 |
# observations and columns
ufo.shape
(80543, 6)
# data structure
ufo.info()
<class 'pandas.core.frame.DataFrame'>
RangeIndex: 80543 entries, 0 to 80542
Data columns (total 6 columns):
# Column Non-Null Count Dtype
--- ------ -------------- -----
0 City 80496 non-null object
1 Colors Reported 17034 non-null object
2 Shape Reported 72141 non-null object
3 State 80543 non-null object
4 Time 80543 non-null datetime64[ns]
5 Year 80543 non-null int64
dtypes: datetime64[ns](1), int64(1), object(4)
memory usage: 3.7+ MB
# numerical summary
ufo.describe()
Year | |
---|---|
count | 80543.000000 |
mean | 2004.178737 |
std | 10.602487 |
min | 1930.000000 |
25% | 2001.000000 |
50% | 2007.000000 |
75% | 2011.000000 |
max | 2014.000000 |
# count the number of ufo reports each year (and sort by year)
ufo.Year.value_counts().sort_index()
1930 2
1931 2
1933 1
1934 1
1935 1
...
2010 4154
2011 5089
2012 7263
2013 7003
2014 5382
Name: Year, Length: 82, dtype: int64
# compare with line plot
ufo.Year.value_counts().sort_index().plot();
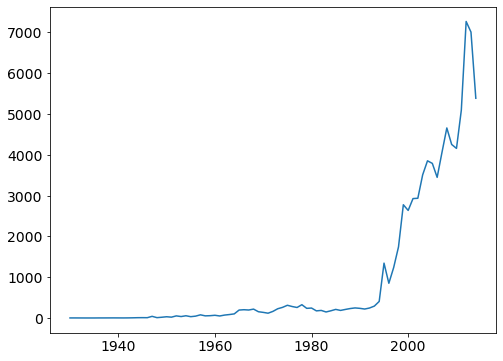
# don't use a line plot when there is no logical ordering
drinks.continent.value_counts().plot(kind='line');
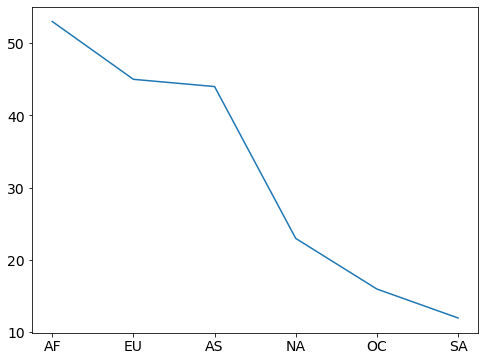
Grouped Box Plots: show one box plot for each group¶
# remainder: boxplot of beer survings
drinks.beer.plot(kind='box');
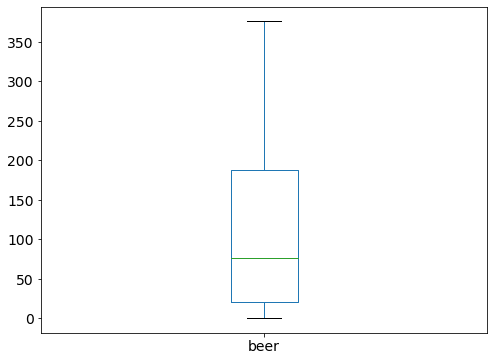
# boxplot of beer survings group by continent
drinks.boxplot(column='beer', by='continent');
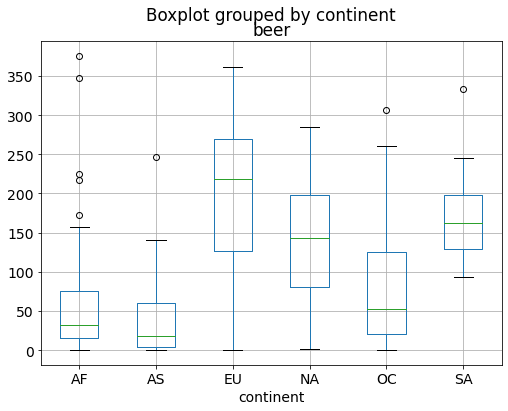
# boxplot of all numerical columns group by continent
drinks.boxplot(by='continent');
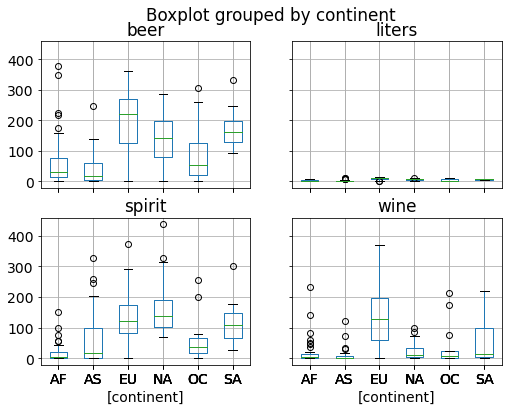
Grouped Histograms: show one histogram for each group¶
# remainder: histogram of beer survings
drinks.beer.plot(kind='hist', bins=20);
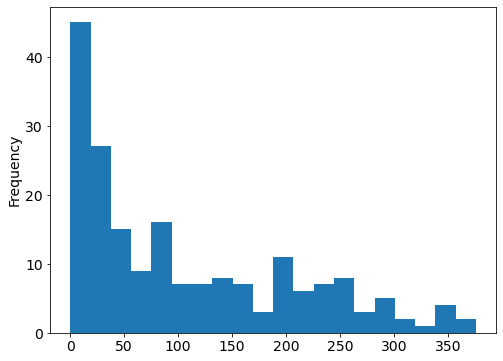
# histogram of beer survings group by continent
drinks.hist(column='beer', by='continent');
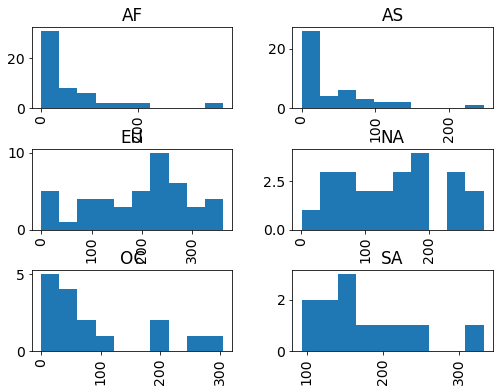
# share the x-axis
drinks.hist(column='beer', by='continent', sharex=True);
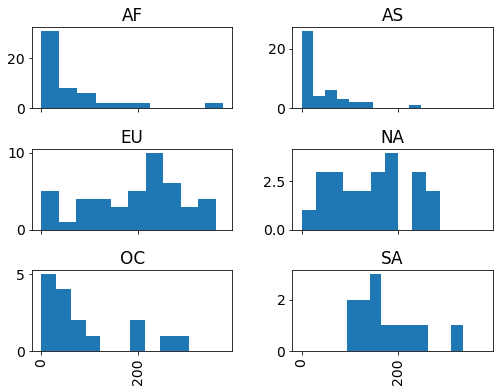
# share the x and y axis
drinks.hist(column='beer', by='continent', sharex=True, sharey=True);
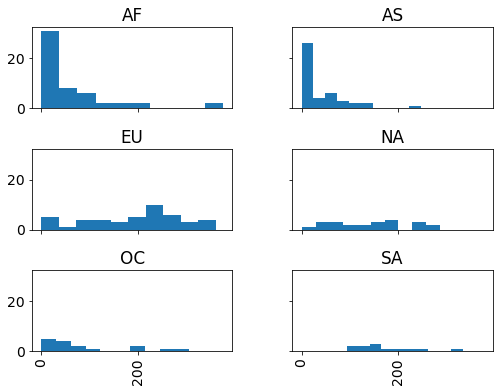
# change the layout
drinks.hist(column='beer', by='continent', sharex=True, layout=(2, 3));
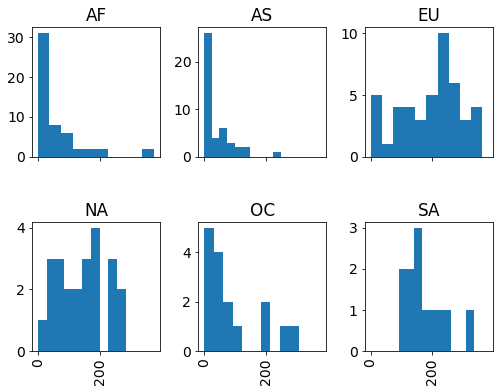
Assorted Functionality¶
# saving a plot to a file
drinks.beer.plot(kind='hist', bins=20, title="Histogram of Beer Survings")
plt.xlabel("Beer Survings")
plt.ylabel("Freequency")
plt.savefig("beer_survings.png") # .png, .tiff, .pdf, .jpeg
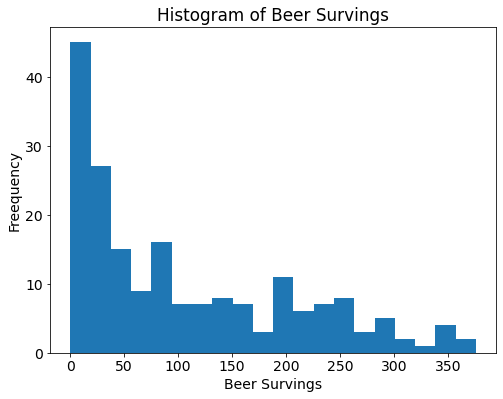
# list available plot style
plt.style.available
['Solarize_Light2',
'_classic_test_patch',
'bmh',
'classic',
'dark_background',
'fast',
'fivethirtyeight',
'ggplot',
'grayscale',
'seaborn',
'seaborn-bright',
'seaborn-colorblind',
'seaborn-dark',
'seaborn-dark-palette',
'seaborn-darkgrid',
'seaborn-deep',
'seaborn-muted',
'seaborn-notebook',
'seaborn-paper',
'seaborn-pastel',
'seaborn-poster',
'seaborn-talk',
'seaborn-ticks',
'seaborn-white',
'seaborn-whitegrid',
'tableau-colorblind10']
# use plot style: ggplot
plt.style.use('ggplot')
# histogram of beer survings in ggplot style
drinks.beer.plot(kind="hist", title="Histogram of Beer Survings")
plt.xlabel("Beer Survings")
plt.ylabel("Frequnecy")
Text(0, 0.5, 'Frequnecy')
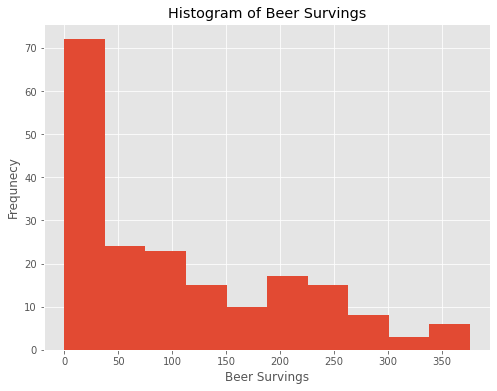
# use plot style: ggplot
plt.style.use('seaborn')
# histogram of beer survings in seaborn style
drinks.beer.plot(kind="hist", title="Histogram of Beer Survings")
plt.xlabel("Beer Survings")
plt.ylabel("Frequnecy")
Text(0, 0.5, 'Frequnecy')
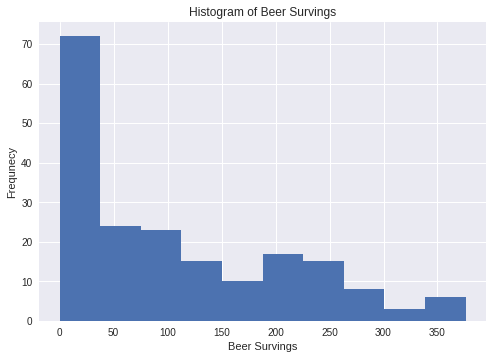
# use plot style: ggplot
plt.style.use('fivethirtyeight')
# histogram of beer survings in fivethirtyeight style
drinks.beer.plot(kind="hist", title="Histogram of Beer Survings")
plt.xlabel("Beer Survings")
plt.ylabel("Frequnecy")
Text(0, 0.5, 'Frequnecy')
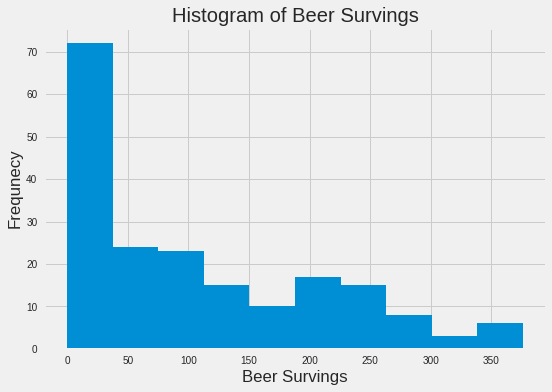